AI risks and the forthcoming regulatory response
Discussions around risks from AI have exploded over the past few years, with contributions from academia 1, civil society 2, management consultancies 3, national authorities 4, as well as international public bodies 5. This has led to a proliferation of different categorisations of the harms that can result from the development, deployment, and widespread use of AI and its various applications. We have synthesised these existing analyses to construct a widely-applicable Risk and Compliance framework. Our framework outlines known risks and harms, but also captures future hazards—unprecedented risks which we may start to encounter as the role of AI in our economy and society continues to expand. We separate risks into three broad categories, based on the pathway through which harm is expected to occur.
In response to extensive and on-going discussions of AI in the public sphere, policymakers are moving swiftly to update emerging technology legislation. This regulatory response is a compelling signal to governance, risk management, and compliance (GRC) functions in many industries around the globe. In this report, we collate a relevant sampling of forthcoming regulations to highlight the compliance requirements that both AI companies and AI-adopting industries must prepare for 6. These requirements tend to be broad—allowing for a variety of interpretations when applied to particular use cases—and they are often applicable to several of our risk categories. As these rules often call for businesses to take precautionary measures, they demonstrate the emerging demand for products and services that can facilitate compliance and best practice risk management.
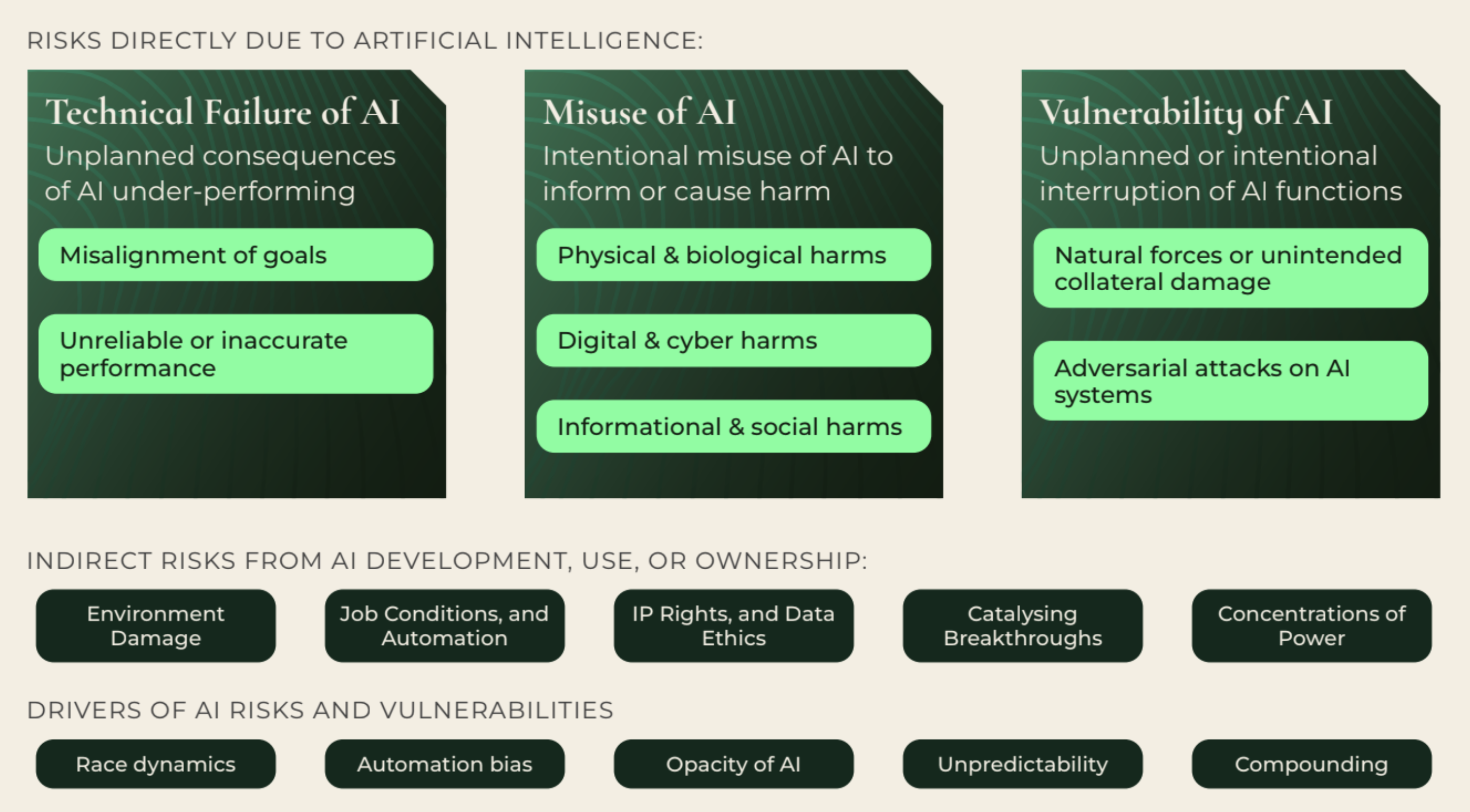
Technical Failure of AI
A Technical Failure is the idea that AI systems themselves may cause harm if they function in a way that no human—whether developer, deployer, or user—intended.
Misalignment of AI
Most AI models we see today are built using a technique called Machine Learning (ML). This technique enables AI systems to learn from input data, develop versatile capabilities, and respond autonomously to situations that were never specified by a human developer. 7 The ability to learn and behave autonomously gives rise to the so-called Alignment Problem, which describes a disconnect between the goals intended by developers of AI systems and the actual outcomes produced by those systems. 8 A misaligned AI system is one that effectively pursues the objective function it was given by humans (e.g., maximising the time social media users spend on a certain platform), but it causes unintended harm in the process. This harm arises either because of a poorly specified objective (e.g., more hours on social media is not equivalent to more enjoyment of social media), or because the instrumental strategy an AI uses to achieve its objective has undesirable side-effects (e.g., maximising time on social media via personalised content feeds that optimise for outrage and fear). These Misalignment risks are of particular concern for increasingly adaptive and autonomous models that learn to make decisions under unfamiliar conditions. This is because complex human values cannot be easily encoded into the language of machines.
As illustrated in the previous paragraph, one well-known example of misaligned AI are widely used social media recommendation algorithms that lead to addiction and the proliferation of hate, fear, and social polarisation. Misalignment also causes problems for the development of safe and reliable AI to use in autonomous vehicles or in coordinating manufacturing processes. While the general goals for AI systems in these applications seem relatively straightforward (e.g., drive safely, smoothly, and efficiently), it is exceedingly difficult to specify those goals comprehensively and in a machine-readable format. If an AI system guided by a narrow set of goals is deployed in a real-life environment, it will systematically discount those human values and goals that were omitted in the algorithm, making decisions that could result in disastrous consequences. For instance, an autonomous “Co-Bot", programmed to minimise production times, could inadvertently cause harm if it did not explicitly recognise and decisively adapt to a young child entering a manufacturing facility. While such a problem might be considered easily solvable (i.e. simply instruct the Co-Bot to not hurt any human encountered), it illustrates a general alignment challenge: it is easy to program an algorithm to avoid clearly specifiable harms, but it is hard to identify and enumerate all the possible ways in which harm can occur. This creates major difficulties for developing AI that remains safe and aligned amidst the many unforeseen situations it is likely to encounter 9.
Example harms from Technical Failure — Misalignment:
Immediate effects | Effects at scale | |
Existing capabilities |
|
|
Potential future capabilities |
|
|
Unreliability of AI
Another characteristic of AI systems that can lead to potentially pervasive and cascading hazards is their unreliable performance. AI systems are known to occasionally provide inaccuracies or fail to complete their given task, whether due to biassed or incomplete input data, technical glitches, or the inherent probabilistic nature of machine learning-based decisions. Depending on the use case and the centrality of AI in the process—ranging from human-in-the-loop informational support to the fully autonomous execution of mission-critical functions—the consequences of unreliable performance vary widely in severity. While an automatically generated ad for an out-of-stock or unrelated product in a supermarket might be annoying at worst, a miscalculation in a system steering a self-driving car can be deadly. Moreover, the sudden malfunctioning of an AI applied to large manufacturing facilities may cause massive economic losses and possibly endanger the lives of factory workers 10.
In addition to these immediately visible failure modes, unreliable performance in AI systems can have corrosive effects that show up only gradually. For instance, biassed systems can inadvertently exacerbate discrimination and thus undermine social justice and cohesion 11, especially if those algorithms are in widespread and prolonged use across the economy. Additionally, incomplete training data can lead to consistently low performance for some groups—e.g., failure to reliably detect and appropriately consider race and gender differences in medical diagnostics 12, or widespread substandard language translations for under-represented languages 13.
Example harms from Technical Failure — Unreliability:
Immediate effects | Effects at scale | |
Existing capabilities |
|
|
Potential future capabilities |
|
|
- Implement measures to address errors, faults or inconsistencies [EU AI Act] 14
- Implement standardised protocols and tools to identify and mitigate systemic risks [EU AI Act]
- Implement mandatory human oversight mechanisms [EU AI Act] [GDPR]
- Provide comprehensive instruction and documentation [EU AI Act]
- Monitor and document incidents [G7 Hiroshima Processes] 15
- Implement robust data governance framework [EU AI Act]
- Record accidents and report to regulatory authorities [UK AI Regulation White Paper] 16
- The EU AI Act, Article 15, prioritises the reliability and robustness of high-risk AI systems, emphasising technical and organisational measures to address errors, faults, or inconsistencies. It also encourages continuous learning post-market deployment to mitigate biassed outcomes.
- Article 55 of the EU AI Act establishes a comprehensive framework for providers of general-purpose AI models with systemic risks 17, involving the implementation of standardised protocols and tools, such as adversarial testing, to actively identify and address systemic risks. Providers are further obligated to evaluate and mitigate these risks at the Union level, keep thorough records of incidents, and promptly communicate pertinent information to regulatory authorities.
- In alignment with Article 14 of the EU AI Act high-risk AI systems require robust human oversight for proper use and to address impacts throughout their lifecycle. Providers must discern appropriate oversight measures, including operational constraints, ensuring assigned individuals possess the necessary competence and authority. Article 14 underscores provider responsibility, emphasising vigilance against automatic reliance on system output, the authority to decide not to use or override system output, and the capability to intervene or halt the system safely. Additionally, in the EU AI Act recital 10 and General Data Protection Regulation (GDPR) 18 recital 71, it is explicitly mentioned that automated data-driven decisions are generally prohibited, with specific exceptions mandated by national legislation 19. Examples of automated data-driven decisions precluded by this legislation may include the automatic rejection of an online credit application and e-recruiting practices without any human intervention. This provision aims to protect individuals from data profiling, ensuring that their rights are not infringed by systems that fail to consider the specific circumstances and context of the individual applicant.
- Furthermore, Article 14 of the EU AI Act finds that transparency requirements are crucial, necessitating high-risk AI systems to be designed for deployers' comprehension of functionality, strengths, and limitations. Accompanying instructions should provide comprehensive information and illustrative examples for enhanced readability. This transparency aids deployers in informed decision-making, correct usage, and compliance. Providers must ensure documentation is meaningful, accessible, and easily understood by target deployers in the language determined by the relevant Member State.
- Objective 2 within the Hiroshima Processes stipulates that organisations should employ AI systems in alignment with their intended purpose, coupled with a commitment to ongoing monitoring for vulnerabilities, incidents, emerging risks, and potential misuse after deployment. An essential facet of this objective encourages organisations to actively facilitate third-party and user engagement in discovering and reporting issues and vulnerabilities post-deployment. Furthermore, organisations are urged to maintain comprehensive documentation of reported incidents, fostering collaborative efforts with relevant stakeholders to effectively mitigate identified risks and vulnerabilities. Mechanisms for reporting vulnerabilities should be easily accessible to a diverse set of stakeholders, promoting transparency and collective responsibility in AI system oversight.
- Article 10 of the EU AI Act outlines requirements for data governance in the development of high-risk AI systems, emphasising the importance of quality training, validation, and testing datasets. These datasets must adhere to appropriate data management practices, consider biases, and be relevant, representative, and error-free for the intended purpose of the model.
- The UK government's February 2024 proposed AI regulatory framework [p.27] emphasises the imperative that AI systems operate in a secure, safe, and robust manner throughout their life cycle, necessitating continuous identification, assessment, and management of potential risks. Recognizing the varying likelihood of AI posing safety risks across sectors, the paper anticipates rigorous regulation in these domains 20.
Misuse of AI
Misuse risks stem from the way in which AI can be used as a powerful tool to facilitate and strengthen attacks against material objects (i.e., physical infrastructure, physical health), digital systems (e.g., personal data, software applications), or informational targets (e.g., online debate fora, personalised communications). In combination or individually, these AI-assisted attacks enhance mal-intentioned (or misguided) actors’ ability to inflict serious political, economic, and social harm.
Physical Harm from Misuse
Risks that fall into the “Physical harm” subcategory are those that result from an ill-intentioned individual or group using AI in an attack against a physical or biological target. Possibly the most prominent example is the use of AI-assisted weapons in violent conflict or the development of bioweapons, which could impact the severity and likelihood of geopolitical confrontation and might change the very nature of war 21. Another risk in this category that has received increasing attention, especially since the release of GPT-3, is the use of AI tools by terrorists and other non-state actors to facilitate the planning and execution of violent attacks. If developers of LLMs fail to implant effective and jailbreak-resistant safeguards, malicious actors might be able to use chatbots and other AI-powered tools to generate instructions for building or acquiring highly destructive weaponry 22. In addition, there is a concern that commercially available autonomous systems (e.g., commercial drones) 23 could be repurposed to execute actions of violence 24, or that dissatisfied workers at a firm developing or deploying AI could get hold of “unconstrained” (free of safeguards) technology and use it for malicious ends.
Immediate effects | Effects at scale | |
Existing capabilities |
|
|
Potential future capabilities |
|
|
Digital Harm from Misuse
Risks that fall into the “Digital harm” subcategory are those that result from an ill-intentioned individual or group using AI in an adversarial cyber operation. This includes attacks to disrupt the data or digital infrastructure of a country, corporation, or other important actor/community, as well as attempts to steal or corrupt digitally-stored information 25. This class of harm escalates the threat level beyond traditional cyber attacks or hacking operations, magnifying both their scale and effectiveness substantially through the use of AI 26. In addition, the “Digital harm” subcategory captures potential harms resulting from surveillance, whether by state entities or private actors 27.
Analogous to attacks against physical targets, risks in this category are concerning because of the massive direct harm they can cause (e.g., if critical infrastructure services such as telecommunications are inhibited, or if swaths of financial customer data are stolen) but also because of indirect effects. For instance, successful adversarial attacks 28 can erode companies’ reputation for reliability and trustworthiness, causing lasting business damage and hampering economic growth. They may also hamper innovativeness as widespread industrial espionage degrades incentives for R&D investments and discourages inventive intellectual property creation 29.
Immediate effects | Effects at scale | |
Existing 30 capabilities |
|
|
Potential future capabilities |
|
|
Informational Harm from Misuse
Artificial intelligence can also be used to create damage that is less tangible than the harm that would result from direct attacks against physical and digital targets. We describe this as “Informational harm,” seeking to capture any intentional use of AI to pollute information environments or to injure individuals’ mental and emotional wellbeing. The difference between digital and Informational harm in our framework lies in the immediate target of an attack: Misuse of AI is classified as “digital” if the application aims to damage digital infrastructures or to corrupt or steal digitised data; the Misuse is classified as “informational” if it explicitly aims to broadly degrade information ecosystems or to feed inaccurate, misleading, or otherwise harmful information to targeted individuals.
Disinformation campaigns, the most prominent risk in this category, can take the form of information warfare directed against the population of a foreign country and state propaganda aimed at a domestic audience 31, but also social engineering targeting companies and their employees 32. Another risk in this category is the intensification of hate speech, which can be produced and spread more quickly through the use of AI, causing emotional anguish and reputational damage to individuals or organisations.
Immediate effects | Effects at scale | |
Existing capabilities |
|
|
Potential future capabilities |
|
|
- Monitor and attest to AI model robustness against misuse [White House Executive Order] 33
- Disclose large-scale cloud computing, and authenticate users [White House Executive Order]
- Identify systemic risks and implement standardised risk mitigation measures [EU AI Act]
- Maintain documentation on risks and report to regulatory authorities [EU AI Act]
- Authenticate content and report on provenance or uncertainty [G7 Hiroshima Processes] [CAC Provisions on Deep Synthesis] 34 [EU AI Act ] [White House Executive Order]
- In the legislative landscape, there is a clear imperative to ensure the robustness of AI models, mitigating the risk of malevolent use. This commitment is highlighted by Section 2 of the White House Executive Order 14110, from 30 October 2023, which emphasises the need for thorough testing and evaluations. These evaluations extend into the post-deployment phase, specifically focusing on performance monitoring, to ascertain that AI systems operate as intended and possess robustness against potential misuse or hazardous modifications 35.
- The proposed rule by the US Department of Commerce titled “Taking Additional Steps To Address the National Emergency With Respect to Significant Malicious Cyber-Enabled Activities” aligns with the directives from the Executive Order, Section 4.2 (c)(i) 36. These documents focus on regulating US infrastructure-as-a-service (IaaS) firms (e.g., Amazon Web Services, Microsoft Azure, Google Cloud), requiring them to report on large AI training runs. It emphasises the necessity for oversight measures to proactively address potential risks and ensure responsible usage of AI technologies in the cloud computing domain. The regulations include a call for the implementation of “Customer Identification Programs” (CIPs), risk-based processes for identity verification and recordkeeping, enhancing oversight and fostering a secure and accountable environment in response to evolving cyber threats and AI misuse 37.
- Similarly, Article 9 of the EU AI Act incorporates a commitment to effective risk management systems, defining them as a continuous, iterative process throughout the entire lifecycle of high-risk AI systems. The Act specifically advocates for the identification and implementation of risk mitigation measures concerning foreseeable misuse within its regulatory purview.
- Article 55 of the EU AI Act provides a detailed framework for providers of general-purpose AI models with systemic risks. This involves adopting standardised protocols and tools, including adversarial testing, to proactively identify and mitigate systemic risks. Providers are also tasked with assessing and mitigating systemic risks at the Union level, maintaining comprehensive incident documentation, and promptly reporting relevant information to regulatory authorities. Ensuring an elevated level of cybersecurity protection for both the AI model and its physical infrastructure is a core aspect of these regulatory obligations.
- In Hiroshima Processes no. 7, 38 emphasis is placed on the development and implementation of robust authentication and provenance 39 mechanisms for AI-generated content, employing techniques such as watermarking 40 to facilitate the identification of the originating AI systems while safeguarding user privacy. Organisations are encouraged to create tools or APIs that allow users to verify the origins of specific content. Additionally, these processes advocate for the adoption of labels or disclaimers to inform users of their interactions with AI systems. Aligning with this, the EU AI Act in Article 50 (4) outlines obligations for providers, particularly those of large online platforms or search engines, to mitigate systemic risks associated with artificially generated or manipulated content, which could adversely affect democratic processes and civic discourse. Similarly, the CAC Provisions on the Administration of Deep Synthesis Internet Information Services mandate that providers conduct technical or manual reviews of AI outputs and implement watermarking and conspicuous labelling if there is potential for public confusion or misinformation. The White House Executive Order 14410 in Section 4.5 (a) calls for the identification and development of science-backed standards, tools, and techniques for authenticating content and establishing robust provenance tracking systems, as well as evaluating methods for labelling synthetic content to enhance transparency and accountability.
Vulnerability of AI systems to exogenous interference
As companies and public services integrate AI systems and these applications become increasingly coupled with business operations, a new class of vulnerability emerges. Risk management for highly relied-upon AI systems becomes imperative to ensure end-to-end processes involving AI are resilient against exogenous interference—whether an unintended disruption, or intentionally-caused breach.
Natural hazards and collateral damage
As AI may be increasingly used to govern critical systems and infrastructure, new kinds of risk due to AI-specific vulnerabilities could result in large-scale catastrophes and cascading consequences. An accidental disruption to AI systems can be the result of a natural hazard (e.g., a flood, an earthquake) or the collateral damage of proximate human-induced attacks (e.g., bombing raids)—i.e., the AI system was not the target, but was caught in the cross-fire and suffered material damage. Though there is not yet any specific regulation mandating measures to reduce vulnerability to these events, public and private actors relying on AI systems for critical functions would do well to take related physical security concerns seriously to prevent costly system breakdowns and their knock-on effects.
Example harms from Vulnerability — Accidents:
Immediate effects | Effects at scale | |
Existing capabilities |
|
|
Potential future capabilities |
|
|
Adversarial attacks
AI systems can be subject to different types of attack, including poisoning attacks (tampering with training data or model algorithms to degrade performance), evasion attacks (tampering with the environment encountered by an AI to cause poor performance in a specific deployment situation), extraction attacks (deducing information about an AI’s training data or about its model architecture through prompt engineering and other strategic interactions with the AI product), and conventional cyberattacks 41. These attacks lead to hazards of data theft (extraction attacks), skewed performance (poisoning and evasion attacks), and system breakdown (conventional attacks), with the attendant consequences in terms of financial, reputational, and human injury as well as negative effects on the business environment, incentives for innovation, and geopolitical tensions 42. As the use of AI becomes increasingly pervasive, these risks grow in scale and severity. This is especially the case if and when AI gets integrated into critical infrastructures, such as transportation and telecommunications systems as well as water and electricity supplies. Moreover, several commentators have argued that AI itself may turn into a general purpose technology that will come to undergird economic and societal systems, which means that it will constitute a critical infrastructure in its own right 43. Vulnerabilities of AI systems will be all the more concerning once this level of AI integration is reached.
Immediate effects | Effects at scale | |
Existing capabilities |
|
|
Potential future capabilities |
|
|
- Design and implement suitable security measures for AI and IT infrastructure [EU AI Act]
- Monitor AI systems for unauthorised alterations, adversarial attacks, and tampering risks [EU AI Act]
- Regularly assess potential risks linked to AI usage in critical infrastructure [White House Executive Order]
- In alignment with the EU AI Act, as articulated in Recital 76, providers of high-risk AI systems are mandated to adopt suitable security measures, encompassing both the AI system and its underlying ICT infrastructure. The recital underscores the dynamic nature of cyberattacks, leveraging AI-specific vulnerabilities like data poisoning, adversarial attacks, or breaches in the digital assets and ICT infrastructure.
- Furthermore, the EU AI Act's Recital 77 calls for a nuanced evaluation of unauthorised attempts to alter the AI system's use, behaviour, or performance. This evaluation encompasses specific AI vulnerabilities such as data poisoning and adversarial attacks, while also acknowledging risks to fundamental rights, as stipulated by the regulation.
- The White House Executive Order Section 4.3 (i) outlines a comprehensive approach to address the risks associated with the deployment of AI in critical infrastructure sectors. These provisions mandate regular assessments of potential risks linked to AI usage in critical infrastructure. These assessments include an examination of how AI deployment might heighten vulnerabilities to critical failures, physical attacks, and cyber threats within these sectors 44.
Footnotes
-
Bernd W. Wirtz, Jan C. Weyerer, and Ines Kehl, “Governance of artificial intelligence: A risk and guideline-based integrative framework”, Government Information Quarterly 39, no. 4 (October 1, 2022), https://doi.org/10.1016/j.giq.2022.101685. ↩
-
Dan Hendrycks, Mantas Mazeika, and Thomas Woodside, “An Overview of Catastrophic AI Risks”, arXiv pre-print (Center for AI Safety, October 2023), https://arxiv.org/abs/2306.12001; and Pegah Maham and Sabrina Küspert, “Governing General Purpose AI — A Comprehensive Map of Unreliability, Misuse and Systemic Risks”, Policy Brief (Stiftung Neue Verantwortung, 20. Juli 2023), https://www.stiftung-nv.de/de/publikation/governing-general-purpose-ai-comprehensive-map-unreliability-misuse-and-systemic-risks. ↩
-
Kevin Buehler et al., “Identifying and managing your biggest AI risks”, Quantum Black. AI by McKinsey, May 3, 2021, https://www.mckinsey.com/capabilities/quantumblack/our-insights/getting-to-know-and-manage-your-biggest-ai-risks. ↩
-
Alina Patelli, “AI: The World Is Finally Starting to Regulate Artificial Intelligence – What to Expect from US, EU and China’s New Laws,” The Conversation, November 14, 2023, http://theconversation.com/ai-the-world-is-finally-starting-to-regulate-artificial-intelligence-what-to-expect-from-us-eu-and-chinas-new-laws-217573. For two concrete examples, see NIST, “AI Risk Management Framework”, Voluntary Guidance (National Institute of Standards and Technology, U.S. Department of Commerce, January 26, 2023), https://www.nist.gov/itl/ai-risk-management-framework; and “Frontier AI: Capabilities and Risks,” Discussion paper (Department of Science, Innovation and Technology (Government of the United Kingdom), October 25, 2023), https://www.gov.uk/government/publications/frontier-ai-capabilities-and-risks-discussion-paper/frontier-ai-capabilities-and-risks-discussion-paper, section: “What risks do frontier AI present?”. ↩
-
UNSG, “Interim Report: Governing AI for Humanity” (AI Advisory Body to the United Nations Secretary-General, December 2023), https://www.un.org/ai-advisory-body. ↩
-
Note: More stringent regulatory requirements will apply where models are above certain [provisionally determined] computing thresholds. These thresholds are measured by the cumulative amount of computational power—or floating point operations (FLOPs)—utilised for training an AI model, and may differ in amount across jurisdictions. Today, the US and EU have set their figures at 1026 and 1025 FLOPs, respectively. It is generally understood that these figures are provisional and are likely to evolve in the coming years. As such, we have not focused herein on demarcating the policies exclusive to today's provisional understanding of powerful general-purpose systems. ↩
-
Jenna Burrell, “How the Machine ‘Thinks’: Understanding Opacity in Machine Learning Algorithms”, Big Data & Society 3, no. 1 (June 1, 2016), https://doi.org/10.1177/2053951715622512. ↩
-
Edd Gent, “What Is the AI Alignment Problem and How Can It Be Solved?”, New Scientist, May 10, 2023, https://www.newscientist.com/article/mg25834382-000-what-is-the-ai-alignment-problem-and-how-can-it-be-solved/. ↩
-
Compare the story of a pedestrian being hit by a self-driving car which had no understanding of the concept of jaywalking, in: Aarian Marshall, “Self-Driving Cars Have Hit Peak Hype—Now They Face the Trough of Disillusionment,” Wired, December 29, 2017, https://www.wired.com/story/self-driving-cars-challenges/. ↩
-
William Thornton, “Alabama Auto Supplier, Agencies Fined $2.5 Million after Woman Crushed,” AL.com (Alabama Media Group), December 14, 2016, https://www.al.com/business/2016/12/alabama_auto_supplier_agencies.html. ↩
-
Brian Hu Zhang, Blake Lemoine, and Margaret Mitchell, “Mitigating Unwanted Biases with Adversarial Learning,” in Proceedings of the 2018 AAAI/ACM Conference on AI, Ethics, and Society, AIES ’18 (New York, NY, USA: Association for Computing Machinery, 2018), 335–40, https://doi.org/10.1145/3278721.3278779. ↩
-
Abeba Birhane, “The Unseen Black Faces of AI Algorithms”, Nature, October 19, 2022, https://doi.org/10.1038/d41586-022-03050-7. ↩
-
Brian Thompson et al., “A Shocking Amount of the Web is Machine Translated: Insights from Multi-Way Parallelism”, arXiv (2024), https://arxiv.org/abs/2401.05749. ↩
-
EU AI Act (def.): EU Act aiming to foster trustworthy AI in Europe and beyond, by ensuring that AI systems respect fundamental rights, safety, and ethical principles and by addressing risks of very powerful and impactful AI models [P9_TA(2024)0138] ↩
-
G7 Hiroshima Processes on Generative AI (def.): International guiding principles on artificial intelligence and a voluntary Code of Conduct for AI developers, prepared for the 2023 Japanese G7 Presidency and the G7 Digital and Tech Working Group, as of 7 September 2023. ↩
-
UK AI Regulation White Paper (def.): proposal of five cross-sectoral principles for existing regulators to interpret and apply within their remits in order to drive safe, responsible AI innovation. [E03019481 02/24] ↩
-
Note: The EU AI Act defines General-Purpose AI Model as “an AI model, including where such an AI model is trained with a large amount of data using self-supervision at scale, that displays significant generality and is capable of competently performing a wide range of distinct tasks regardless of the way the model is placed on the market and that can be integrated into a variety of downstream systems or applications, except AI models that are used for research, development or prototyping activities before they are released on the market.” The Act distinguishes a GPAI Model from a General-Purpose AI System, which is described as “an AI system which is based on a general-purpose AI model, that has the capability to serve a variety of purposes, both for direct use as well as for integration in other AI systems.” ↩
-
General Data Protection Regulation (def.): comprehensive data protection law enacted by the European Union to safeguard individuals' personal data and regulate its processing by organisations [Regulation (EU) 2016/679] ↩
-
Regulation (EU) 2016/679 of the European Parliament and of the Council of 27 April 2016 on the protection of natural persons with regard to the processing of personal data and on the free movement of such data, and repealing Directive 95/46/EC (General Data Protection Regulation) (GDPR) [2016] OJ L 119/1, recital 71. ↩
-
Michelle Donelon, “A Pro-Innovation Approach to AI Regulation: Government Response,” Consultation Outcome presented to Parliament by the Secretary of State for Science, Innovation and Technology by Command of His Majesty (Department of Science, Innovation and Technology (Government of the United Kingdom), February 6, 2024), https://www.gov.uk/government/consultations/ai-regulation-a-pro-innovation-approach-policy-proposals/outcome/a-pro-innovation-approach-to-ai-regulation-government-response. ↩
-
Paul Scharre, Army of None: Autonomous Weapons and the Future of War (W. W. Norton & Company, 2018). ↩
-
Jonas Sandbrink, “ChatGPT Could Make Bioterrorism Horrifyingly Easy”, Vox, August 7, 2023, https://www.vox.com/future-perfect/23820331/chatgpt-bioterrorism-bioweapons-artificial-inteligence-openai-terrorism; and Thomas Gaulkin, “What Happened When WMD Experts Tried to Make the GPT-4 AI Do Bad Things,” Bulletin of the Atomic Scientists, March 30, 2023, https://thebulletin.org/2023/03/what-happened-when-wmd-experts-tried-to-make-the-gpt-4-ai-do-bad-things/. ↩
-
Note: Beyond making AI models and tools available on the market, AI developers or deployers may inadvertently enable the Misuse of AI for physical harm if they fail to sufficiently secure their systems against theft. That could allow malicious actors to steal AI models or applications and then use them for harmful ends. For more details on risks related to AI systems’ vulnerability to adversarial attacks, see below (“Adversarial attacks: exploiting AI system vulnerabilities intentionally”). ↩
-
Miles Brundage et al., “The Malicious Use of Artificial Intelligence: Forecasting, Prevention, and Mitigation” (arXiv, February 20, 2018), https://doi.org/10.48550/arXiv.1802.07228, pp. 27-28, 37-43. ↩
-
Note: The “Digital Harm” sub-category does not include the use of AI for spreading disinformation or hate speech—i.e., acts that intend to degrade our shared information commons. This type of AI misuse is separately classified into the “Informational Harm” sub-category. We acknowledge that often no distinction is made, given Informational Harms from AI are propagated through digital means; however, our choice reflects that a distinct risk management response may be required for digital and informational harms. ↩
-
MIT Technology Review Insights und Darktrace, “Preparing for AI-Enabled Cyberattacks”, MIT Technology Review, April 8, 2021, https://www.technologyreview.com/2021/04/08/1021696/preparing-for-ai-enabled-cyberattacks/; “Frontier AI: Capabilities and Risks,” Discussion paper (Department of Science, Innovation and Technology (Government of the United Kingdom), October 25, 2023), https://www.gov.uk/government/publications/frontier-ai-capabilities-and-risks-discussion-paper/frontier-ai-capabilities-and-risks-discussion-paper, section: “What risks do frontier AI present?”; and Lothar Fritsch, Aws Jaber, and Anis Yazidi, “An Overview of Artificial Intelligence Used in Malware,” in Nordic Artificial Intelligence Research and Development, ed. Evi Zouganeli et al., Communications in Computer and Information Science (Cham: Springer International Publishing, 2023), 41–51, https://doi.org/10.1007/978-3-031-17030-0_4. ↩
-
Simon McCarthy-Jones, “Artificial Intelligence Is a Totalitarian’s Dream – Here’s How to Take Power Back,” The Conversation, August 12, 2020, http://theconversation.com/artificial-intelligence-is-a-totalitarians-dream-heres-how-to-take-power-back-143722; and Pegah Maham und Sabrina Küspert, “Governing General Purpose AI — A Comprehensive Map of Unreliability, Misuse and Systemic Risks”, Policy Brief (Stiftung Neue Verantwortung, 20. Juli 2023), https://www.stiftung-nv.de/de/publikation/governing-general-purpose-ai-comprehensive-map-unreliability-misuse-and-systemic-risks. ↩
-
Note: AI systems can be subject to different types of attack, including poisoning attacks, evasion attacks, extraction attacks, and conventional cyberattacks. For more information, refer to the subsection "Adversarial attacks" below. ↩
-
European Commission (Directorate-General for Internal Market, Industry, Entrepreneurship and SMEs) and PwC, “The Scale and Impact of Industrial Espionage and Theft of Trade Secrets through Cyber” (Publications Office of the European Union, 2018), https://data.europa.eu/doi/10.2873/48055, p. 27. ↩
-
When it comes to the use of AI in cyber attacks, experts are not certain what exactly current models are capable of and how these capabilities stack up against existing defensive measures. There is also no guaranteed knowledge on which cyber operations AI might have been used for already. For instance, compare “Frontier AI: Capabilities and Risks,” Discussion paper (Department of Science, Innovation and Technology (Government of the United Kingdom), October 25, 2023), https://www.gov.uk/government/publications/frontier-ai-capabilities-and-risks-discussion-paper/frontier-ai-capabilities-and-risks-discussion-paper, section: “What risks do frontier AI present?”; and Lothar Fritsch, Aws Jaber, and Anis Yazidi, “An Overview of Artificial Intelligence Used in Malware,” in Nordic Artificial Intelligence Research and Development, ed. Evi Zouganeli et al., Communications in Computer and Information Science (Cham: Springer International Publishing, 2023), 41–51, https://doi.org/10.1007/978-3-031-17030-0_4. ↩
-
Tate Ryan-Mosley, “How Generative AI Is Boosting the Spread of Disinformation and Propaganda”, MIT Technology Review, October 4, 2023, https://www.technologyreview.com/2023/10/04/1080801/generative-ai-boosting-disinformation-and-propaganda-freedom-house/. ↩
-
Vincenzo Ciancaglini et al., “Malicious Uses and Abuses of Artificial Intelligence” (Trend Micro Research, United Nations Interregional Crime and Justice Research Institute (UNICRI), Europol’s European Cybercrime Centre (EC3), June 12, 2021), https://www.europol.europa.eu/publications-events/publications/malicious-uses-and-abuses-of-artificial-intelligence. ↩
-
White House Executive Order 14110 (def.): United States Executive Order promoting the development and implementation of repeatable processes and mechanisms to understand and mitigate risks related to AI adoption, especially with respect to biosecurity, cybersecurity, national security, and critical infrastructure risk [88 Federal Register 75191]. ↩
-
CAC Provisions on Deep Synthesis (def.): Chinese provisions to regulate the use of deep synthesis technologies, AI-based technologies used to generate text, video, and audio, also known as deepfake. The Provisions prohibit the generation of “fake news” and require synthetically generated content to be labelled. ↩
-
Executive Office of the President, “Safe, Secure, and Trustworthy Development and Use of Artificial Intelligence” (88 Federal Register 75191, November 1, 2023), Section 2(a), https://www.federalregister.gov/documents/2023/11/01/2023-24283/safe-secure-and-trustworthy-development-and-use-of-artificial-intelligence. ↩
-
United States Department of Commerce. "Taking Additional Steps to Address the National Emergency With Respect to Significant Malicious Cyber-Enabled Activities." Federal Register 86, no. 184 (September 24, 2021): 53721-53726. https://www.federalregister.gov/documents/2021/09/24/2021-20430/taking-additional-steps-to-address-the-national-emergency-with-respect-to-significant-malicious ↩
-
Nicholas Welch, “Can BIS Control the Cloud?,” ChinaTalk, January 31, 2024, https://www.chinatalk.media/p/can-bis-control-the-cloud. ↩
-
OECD, “G7 Hiroshima Process on Generative Artificial Intelligence (AI): Towards a G7 Common Understanding on Generative AI,” Report prepared for the 2023 Japanese G7 Presidency and the G7 Digital and Tech Working Group (Paris: OECD Publishing, September 7, 2023), https://doi.org/10.1787/bf3c0c60-en. ↩
-
Content Provenance (def.): Knowledge of the origins, history, ownership, and authenticity of media. Facilitated through documentation, it establishes the source of content and whether it may have undergone alterations over time. Provenance is crucial for verifying the integrity and ownership of media in digital or online environments. [The Royal Society] ↩
-
Watermarking (def.): "A way to identify the source, creator, owner, distributor, or authorised consumer of a document or image. Its objective is to permanently and unalterably mark the image so that the credit or assignment is beyond dispute" [Berghel H., et al] ↩
-
Note: Some authors also discuss the misuse of AI systems for malicious purposes. This is one valid approach for categorising risks from AI, but it differs from the logic underlying our framework, where any malicious use of AI systems, whether or not intended by its developers, falls into the Misuse category. ↩
-
Apostol Vassilev et al., “Adversarial Machine Learning: A Taxonomy and Terminology of Attacks and Mitigations” (National Institute of Standards and Technology, U.S. Department of Commerce, NIST AI 100-2 E2023, January 4, 2024), https://doi.org/10.6028/NIST.AI.100-2e2023; BSI, “AI Security Concerns in a Nutshell - Practical AI-Security Guide” (Bonn: Federal Office for Information Security, German Federal Government, March 9, 2023), https://www.bsi.bund.de/SharedDocs/Downloads/EN/BSI/KI/Practical_Al-Security_Guide_2023.html. ↩
-
Yu, Chen. AI as critical infrastructure: Safeguarding National Security in the age of Artificial Intelligence, 2024. https://doi.org/10.31219/osf.io/u4kdq. ↩
-
Executive Office of the President, “Safe, Secure, and Trustworthy Development and Use of Artificial Intelligence” (88 Federal Register 75191, November 1, 2023), Section 4.3 (i), https://www.federalregister.gov/documents/2023/11/01/2023-24283/safe-secure-and-trustworthy-development-and-use-of-artificial-intelligence. ↩